AI systems have a profound impact on many lives. And yet the technology industry largely lacks the auditing, evaluation, and accountability mechanisms that are common in other industries.
That needs to change. It’s time to invest in the tools we need to hold algorithms accountable.
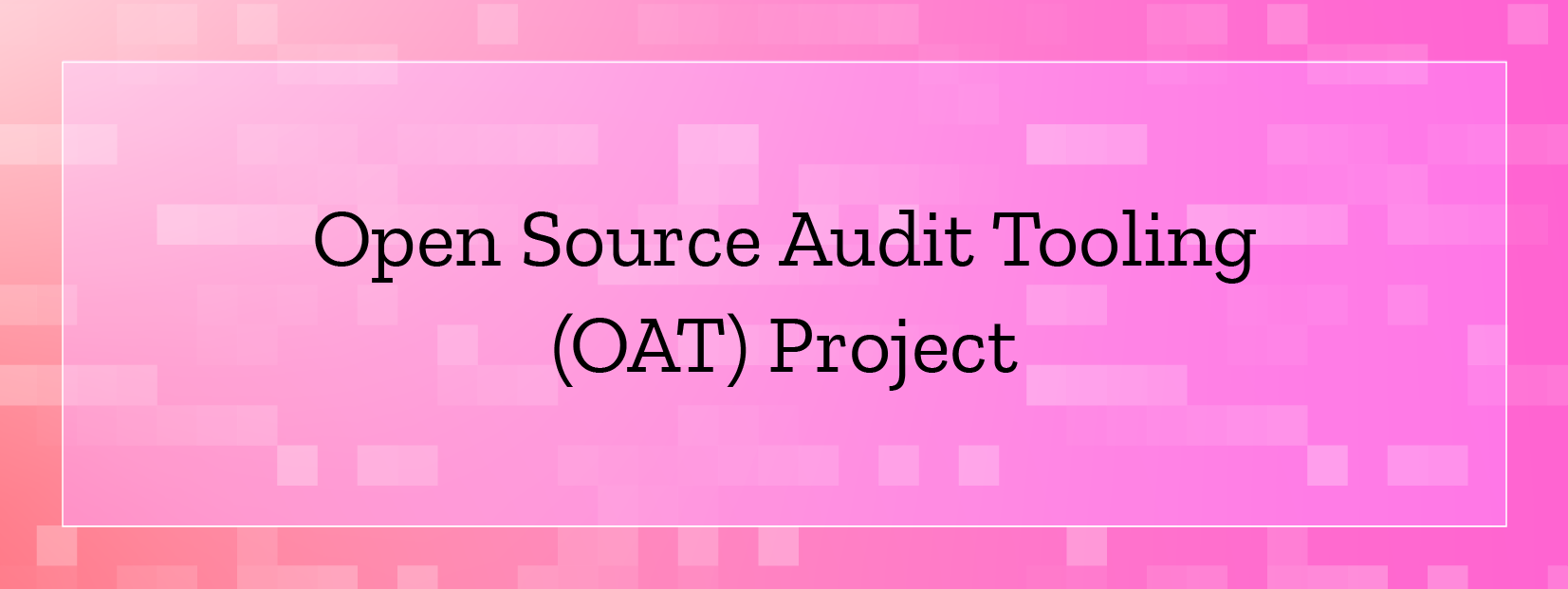
Overview
The cars we drive face rigorous crash and emissions tests. The food we eat is subject to careful inspections. And yet the algorithms in our lives — which influence everything from who qualifies for a loan to who gets parole — face little dedicated, formal scrutiny.
When these algorithms fail, people get hurt: false arrests and wrongful accusations; glitches blocking access to healthcare or housing; and biased outcomes creating barriers for the most vulnerable to succeed.
Evidence of these failures, biased outcomes and consequential harms has been collected for years now by algorithmic auditors, who meticulously analyze AI systems. However, algorithmic audits are still surprisingly ad hoc, often developed in isolation of other efforts and in the presence of meager resources.
That’s why, over the coming year, Mozilla Fellow Deb Raji is running the Open Source Audit Tooling (OAT) Initiative. Deb will identify the resources and tools needed to support algorithmic auditors, and to make thorough and consequential AI scrutiny the status quo.
The research will be guided by two central questions: What are the tools and resources auditors need in order to be successful in accomplishing their goals? And how do we incentivize and build these tools?
In this work, the focus is specifically on open source tooling, given its historical role in not just resourcing individual and institutional stakeholders, but also supporting community growth and maturation through the consolidation of norms, shared vocabulary and objectives.
Timeline
Phase 1 | Open Source Audit Tools Survey and Taxonomy
In this phase, we will articulate the need for algorithmic audit tools and gaps in audit tool development; map out the known design space for audit tool development in AI and other industries; develop a taxonomy of considerations; and map out categories of tools and the needed resources for development for these tools.
Phase 2 | Exploration of Interventions for Improved Audit Tool Development
In this phase, we will brainstorm potential interventions to address gaps in effective audit execution and tool development; assess the value of doing a public challenge to promote open-source development of algorithmic auditing tools and benchmarks; and map out opportunities for Mozilla to get involved in auditing space and operate a hub for open source audit tools and datasets.
Phase 3 | Implementation of Prioritized Intervention for Audit Tool Development
In this phase, we will plan and launch audit challenges and/or product development, invite participants to engage in an implemented solution; and launch workshop meetings with key audit tool development stakeholders.
Get Involved
We are seeking contributors who share our principals — from engineers, to activists, journalists, legal experts, and beyond. You can help inform, guide, and conduct our research.
Specifically, we are seeking:
Audit practitioners and tool developers who build and work with AI auditing tools regularly. You may be a journalist, civil society researcher, data scientist, regulator or academic — if you’ve participated in AI audits, used or developed a tool you think we should know about, please let us know! We would love to hear about your experiences and factor this into our research.
Audit participants who have familiarity with this space and want to work in the future as a team member or research assistant.
If you want to get involved or learn more, please reach out directly to [email protected].
News
It’s Time to Develop the Tools We Need to Hold Algorithms Accountable
Published by Deb Raji on February 2, 2022
Related Audit Work at Mozilla
This work ties into a set of related projects happening at Mozilla regarding algorithmic audit tooling, some of which is listed below.
Mozilla Technology Fund supports open source technologists whose work furthers promising approaches to solving pressing internet health issues.
Regrets Reporter, a project for crowdsourced analysis of YouTube's recommendation algorithm.
Mozilla Rally, a tool for users to donate data to uncover Facebook’s tracking network, understand search engine choice and help local news find sustainability.
Glossary
Helpful context and definitions.
Audits are evaluations with an expectation for accountability (i.e. an informed and consequential judgment for the actions of decision-making actors). Note that audits can be for assessing bias or functionality issues but can also go beyond that to evaluate any type of potential harm, including security, privacy, and other safety issues.
Audit tools are any resource that support algorithmic analysis and inspection (including benchmarks/datasets, analysis tools, documentation templates, etc.). These tools may support the assessment of expectations institutions have of themselves as well as expectations others have of them, at various stages of design and development (i.e. pre- and post- deployment).
Internal auditors seek to validate procedural expectations, aim to minimize liability and test for compliance to AI principles and legal constraints. They are often employees or contractors of the audit target.
External auditors aim for a material change in the situation (i.e. product update, policy change, recall, etc.) to minimize the harm being experienced by those they represent. They often have no formal contractual relationship with the audit target.