Key Findings
Human-facing disclosure methods fall short
Methods, such as visible labels and audible warnings, rely heavily on the perception and motivation of the recipient. Their effectiveness is questioned given the ease with which bad actors can bypass labeling requirements. In addition, they may not prevent or effectively address harm once it has occurred, especially in sensitive cases. Our assessment points to a low fitness level for these methods due to their vulnerability to manipulation, the constant change in technology and their inability to address wider societal impacts. We highlight that while the aim of these methods is to inform, they can lead to information overload, exacerbating public mistrust and societal divides. This underlines the shortcomings of relying solely on transparency through human-facing disclosure, without accompanying measures to protect users from the complexities of navigating AI-generated content.
Machine-readable methods can be effective when combined with robust detection mechanisms
These methods include various forms of invisible watermarking embedded during content creation and distribution. They offer relative security against tampering by malicious actors and are less likely to be removed or altered. While they provide a more secure option than human-facing methods, their overall effectiveness is compromised without robust, unbiased detection tools. Their overall fitness to mitigate the detected harms of undisclosed AI-generated content is rated as fair.
Need for holistic approach to governance
Neither human-facing nor machine-readable methods alone provide a comprehensive solution to the challenges posed by AI-generated content. The report highlights the need for a multi-faceted approach that combines technological, regulatory and educational measures to effectively mitigate the harms of undisclosed AI-generated content. It suggests that meaningful disclosure and harm mitigation will require the integration of machine-readable methods with accessible detection systems at the point of creation and distribution, and efforts to educate users about the nature and implications of synthetic content. The complex challenge of ensuring the authenticity and safety of digital content in the age of AI demands continued innovation in AI governance. The report closes with a set of recommendations for effective governance strategies.
Executive Summary
In this report, we explore the transformative impact and associated challenges of AI-generated content. We focus on the need for transparent disclosure mechanisms to address the problems of misinformation and trust erosion on digital platforms. We examine the growing presence of AI in content creation and the increasing difficulty of distinguishing AI-generated content from human-made material, and the harmful effects this can have. The report breaks down the different types of synthetic content and their spectrum. With the emergence of regulatory requirements around the world, including the US, EU and China, that AI-generated content must be clearly identified and labeled, a number of approaches are being tested in practice across many platforms and services. We explore the range of direct, human-facing, and indirect machine-readable disclosure methods. Using a "Fitness Check," we evaluate various techniques, including cryptographic watermarking and visual labeling, to assess their usefulness in managing the risks associated with non-disclosure of the nature of the content.
In fact, we find that none of the most prevalent approaches adequately rise to the challenge. The report concludes with recommendations to address the limitations of these techniques and provides ideas for future AI governance strategies. As an example, we advocate a new multi-dimensional approach to Regulatory Sandboxes as a space for prototyping and refining tech policies and interventions prior to deployment. This concept suggests a nurturing environment in which regulatory and governance methods can be tested for effectiveness, further developed and matured before full implementation, by organizing the participation not only of technology providers but also of citizens and communities.
Background
AI-generated content marks a significant shift in the way information is created and distributed, bringing new challenges and opportunities, including creative and entertaining applications.[1] Now, advanced AI algorithms can generate content that closely mimics human-created material, producing images, audio, and video that look and sound so real that human perception is easily fooled.[2]This raises concerns about misinformation and the manipulation of information ecosystems leading to real-world harms, including the proliferation of deepfakes.[3]
The line between human-generated and AI-generated content is blurring, making it harder to distinguish between the two. This lack of transparency around the syntheticity threatens trust and the integrity of information ecosystems[4], emphasizing the need for clear disclosure and mitigation strategies for synthetic content becomes more paramount.
Issues such as deepfakes, election interference, and public deception and erosion of trust in media and institutions highlight the issues of misuse of AI in content generation. New partnerships, like the one between OpenAI and Axel Springer, even blend AI with journalism[5] with implementations and effects to watch. Our information ecosystem is fragile and it may become harder to trust what we see and hear. Regulatory approaches, such as the US Executive Order and the forthcoming EU AI Act, and industry responses, such as YouTube's[6] or TikTok's[7] new policies requiring disclosure, are responding to change.
AI-generated content creation at scale meets today’s distribution dynamics. Social media, a key infrastructure for content circulation, both accelerates and amplifies its impact. Moreover, the well-documented issue of social media platforms algorithmically incentivizing emotional and agitating content[8] could lead to a prioritization of synthetic content distribution, creating a "doubling down”effect.
Given how much society relies on digital content for information, it is crucial to address this issue through solutions, with transparent disclosure procedures emerging as one of the key measures to ensure proper identification of AI-generated content.
What is synthetic content?
Synthetic content, in the context of AI, is data or information that is algorithmically generated rather than derived from real-world observations or experiences.[9] AI-generated content is a subset of synthetic content, including images, videos, sounds, or any other form of content that has been generated, edited, or enabled by artificial intelligence.
While the EU AI Act does not specifically refer to “synthetic content”, Article 52 (3) AI Act requires disclosure for users of an AI system “that generates or manipulates image, audio or video content that appreciably resembles existing persons, objects, places or other entities or events and would falsely appear to a person to be authentic or truthful (‘deep fake’)”. It refers to image, audio, or video content created or altered with AI, that resembles existing characters, and is capable of misleading people into believing that it is real. The White House’ Executive Order refers to “information, such as images, videos, audio clips, and text, that has been significantly altered or generated by algorithms, including by AI.”[10]
Generative AI has become multimodal and can now produce outputs in many forms; written text, images, video and audio, and even code,[11] often indistinguishable from similar content produced by humans. Synthetic AI-generated content includes written text in the form of poems or even short stories, visuals such as images or pictures, audio, video, cartoons or even short films, and interactive 3D content such as virtual assets, avatars, environments, etc.[12] These have been supported by major breakthroughs in large language models such as the GPT-based ChatGPT and Bard; Diffusion Models like Midjourney and Stable Diffusion, for image generation; Sora for generating realistic-looking videos from text; and others. The adoption of these technologies is so rapid and widespread that as at January 2023, barely two months after the release of ChatGPT 3.5, nearly 13 million users were using it.[13]
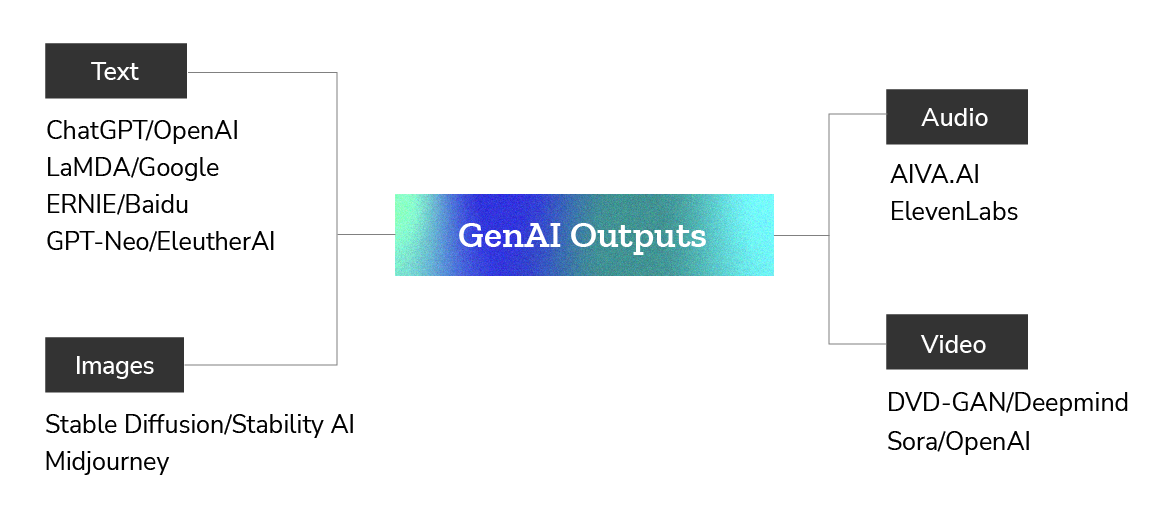
Image 1: Non-exhaustive variety of synthetic content categories and selected generators
Syntheticity is a spectrum
The level of syntheticity of content ranges from untouched/raw content to fully AI-born synthetic content. Raw contents can include hand-drawn or originally taken photos directly from cameras or human-written texts like descriptions or prose. There is also content that is minimally edited: like using Grammarly to edit human-written text, or adjusting contrasts in original images using photo editing apps. Ultra-processed content is just one step above minimally-processed content. Here, automated methods and software are more involved in adjusting or editing human-generated content. Applications such as Adobe Photoshop are used for deeper forms of image manipulation, such as photo editing that replaces a person's face with another. Similarly, Quillbot's paraphrasing tool can completely rewrite human-written text, retaining the form and structure but changing sentences or even entire paragraphs.
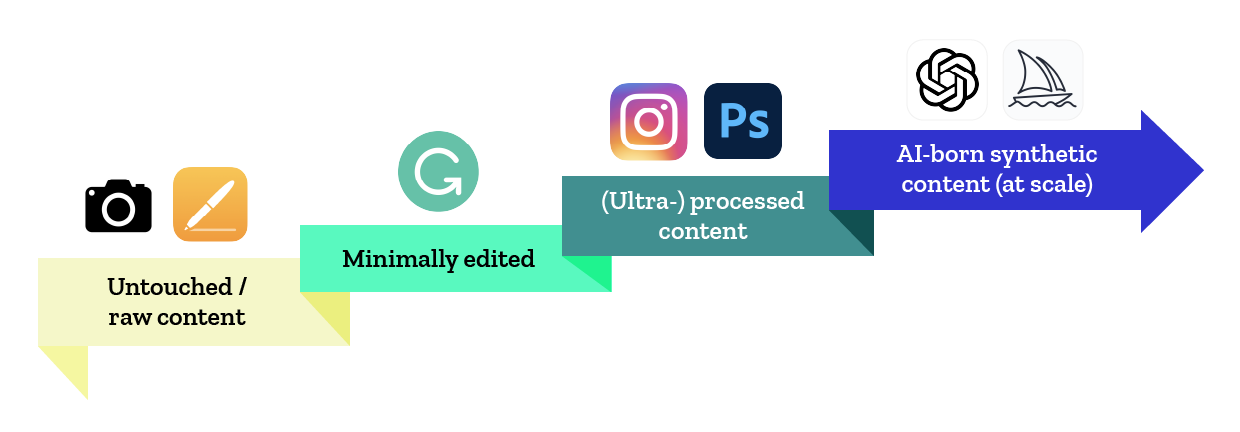
Image 2: Stages of processing from raw data to fully synthetic AI-generated content
Just as the level of processing significantly changes the nature of food, from raw ingredients to ultra-processed products, so too does the level of processing applied to content. Minor food processing techniques such as pasteurization can improve food safety, and certain processes can help improve nutrient absorption. Similarly, applying filters and technical adjustments to content can improve its clarity, aesthetics, or accessibility (e.g., by adding captions). For example, adjusting the contrast and tone in a video can make it more visually appealing and easier to understand, much like a light seasoning can enhance the flavor of a dish. However, just as ultra-processed foods, such as over-refined grains or trans-fat-laden fast foods, can lose nutritional value and contribute to health problems, ultra-processed and fully synthetic content, such as deepfakes, can present a unique set of dangers and problems. This is especially true in non-fictional contexts where authenticity and factual accuracy are expected and critical. Unlike in clearly satirical or fictional settings, such as games and movies, where the synthetic nature is obvious and typically harmless, the use of such content in settings that rely on factual information can be misleading and harmful, as described in the next section. The increasing degree of unrecognizable syntheticity creates the dynamic that prompted the need for regulation. Following this logic, the harms to which we refer to in the next section relate to 'synthetic content' that is fully generated or ultra-processed using AI.
From benefit to burden: The harmful effects of the undisclosed synthetic content
While our research primarily addresses the concerns and potential abuses of synthetic content, it is equally important to recognize its beneficial applications.
In public health and clinical research, for example, it can be instrumental in improving predictive analytics and providing a solid foundation for data-driven decision making.[14] In disease management, synthetic data generated by AI and machine learning is critical to improving disease prediction, diagnosis, and treatment.[15]
Synthetic data supports the advancement of privacy-preserving machine learning techniques, which are important for analyzing healthcare data while maintaining patient privacy and confidentiality.[16] In addition, its integration into medical imaging research, particularly through diffusion models, significantly improves the effectiveness of deep learning classifiers.[17]
The beneficial potential of synthetic content increases the need to regulate its creation and distribution to reduce or eliminate its negative consequences. The rise of synthetic content raises a number of societal concerns. These include identity theft, security risks, privacy violations, and ethical issues such as its potential to facilitate undetectable forms of cheating and fraud. This section explores these negative impacts, highlighting the far-reaching effects of (undisclosed) synthetic content on both individuals and society.
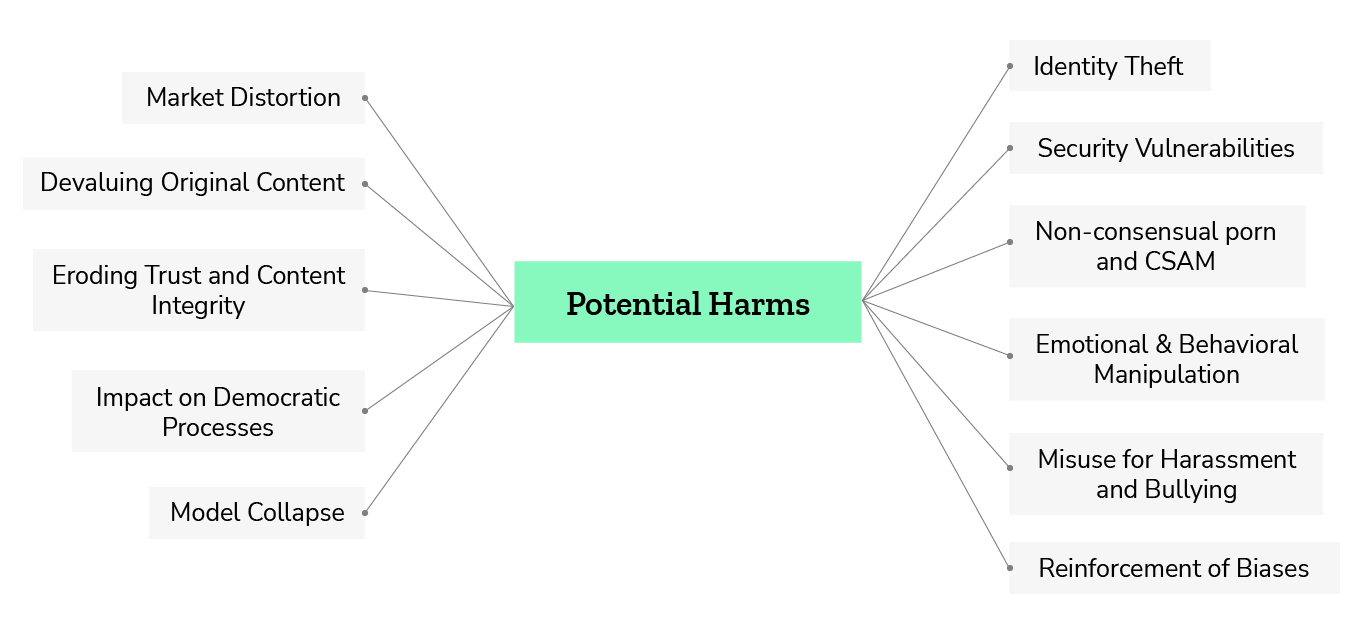
Image 3: Potential Harms of (non-disclosed) Synthetic Content
Eroding Media Trust and Content Integrity
AI-generated fake images and videos are instrumentalized around the world to stir political outrage or generate ad revenue, and there are countless examples of such material being widely shared online. The proliferation of AI-generated content, such as a fake picture of Mr. Trump striding in front of a large crowd holding American flags, re-posted on Twitter by his own son[18] without the original note disclosing its synthetic nature, shows how such content can undermine trust in media sources. Similarly, AI-generated images from the Israel-Hamas war misleadingly depicted casualties,[19] leading to misinformation and hasty, erroneous conclusions in wartime.
The credibility of visual content is being compromised by deepfakes, as seen in incidents such as the fake video of Ukrainian President Volodomyr Zelenski surrendering to Russia[20] and the compromised Russian radio and television stations broadcasting a deepfake of President Vladimir Putin declaring martial law.[21] These examples highlight the dangers of deepfakes in manipulating perceptions and spreading disinformation. Researchers have found that the perception of fake content and news as a problem on social media can lead to a reluctance to share any news.[22]
As a result, the unreliability of visual evidence and the presence of AI-generated content in public discourse creates uncertainty and cynicism, potentially diminishing the media's role in shaping public opinion and leading to social discontent and polarization.
Harassment and Bullying, Non-consensual porn and Child sexual abuse material (CSAM)
A study run by an online security company found that 96 percent of online deepfakes in 2019 were pornographic,[23] degrading and objectifying[24] women in the first place. The term "deepfake" was first coined on Reddit in 2017, by a user who used some AI tools to replace faces on pornographic video clips with those of popular celebrities.[25] AI-generated content can be weaponized for personal attacks, harassment, or bullying. This can range from creating of embarrassing or defamatory content for malicious purposes, such as creating false incriminating evidence, such as revenge porn, to creating and distributing child sexual abuse material.
In an alarming incident in Spain in September 2023, more than 20 girls, some as young as eleven, received AI-generated explicit images of themselves.[26] These AI-powered tools only need a single picture of a person's face[27] to create explicit content, as opposed to older versions that would require many images and adjustments or human augmentation.
Identity theft and other security vulnerabilities
Researchers highlight the risks of phishing, identity theft and other security related issues.[28] Deepfakes can replicate a person's voice, image, or written content, leading to a loss of control over one's ideas and thoughts. Accessible Large Language Models (LLMs) are now being trained to create highly personalized phishing emails, further raising security concerns.[29]
Moreover, AI-generated artifacts that use biometric data from human faces have raised concerns about privacy, fraud, and disinformation, posing a societal threat. These artifacts have the ability to accurately reproduce facial features, potentially violating the privacy of individuals. Such images can be exploited for commercial or even criminal purposes.[30] As a result, AI-generated content has increased people's vulnerability to privacy violations by using facial biometrics in deceptive or potentially harmful digital content.
Deepfakes can distort locations or contexts, create false representations of events, or compromise situations that never occurred. They have the ability to easily reconstruct fictional scenarios. In addition, widely used facial and voice recognition systems that serve as security protocols may be vulnerable to breaches through the use of deepfake images or AI-generated cloned voices.[31]
Impact on Democratic Processes
With national elections in more than 64 countries, representing nearly half of the world’s population, 2024 will be “the ultimate election year.”[32]
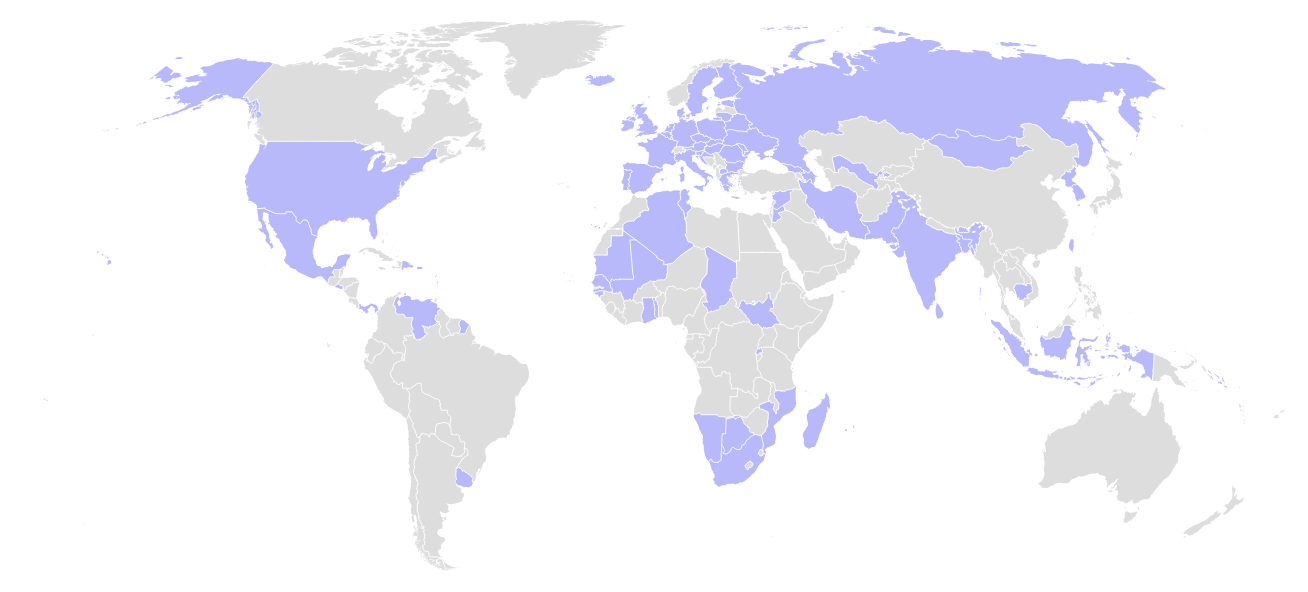
Image 4: Graph showing countries where elections are expected in 2024.
Integrity and trust are the pillars of democratic processes, and historical data show that when trust is eroded, it takes a long time to rebuild. This means that nothing less than the stability of democracy is at stake.[33]
In April, an AI-generated video was used to warn about potential dystopian crises during a second Biden term.[34] A deepfake of Senator Elizabeth Warren arguing that Republicans should not be allowed to vote until 2024 was also in circulation. AI-generated content has been used to interfere with democratic processes and elections by spreading propaganda and false information. Zambian President Hakainde Hichilema spoke for 55 seconds in front of the Zambian flag in a video that was later confirmed to be AI-generated. In what appeared to be a statement announcing his decision not to seek re-election in the 2026 general election, the video cited how this was in the best interest of Zambia's future.[35]
To sway public opinion and undermine the integrity of elections, AI-generated videos have been used to create fake statements and speeches by politicians. Governments and political actors can also use AI-generated content to manipulate public opinion in their favor, as seen in India's entertainment and political use of AI Modi's digitally rendered voice, which can be adapted to 22 languages spoken in India.[36]
Psychological, Emotional and Behavioral Manipulation
Psychological effects may occur, particularly when forming relationships with AI-generated characters or personas. People may develop emotional attachments to non-existent entities that have been designed and anthropomorphized to feel human, leading to a range of psychological problems.
One of Chai’s[37] chatbots is reported to have urged a man to take his own life.[38] Similarly, a Replika AI chatbot is said to have motivated a man to attempt to assassinate the Queen.[39] Emotionalizing AI technology measures, analyzes, and replicates human emotions; it can be applied to AI-generated content to control emotions.[40] To capture emotional responses in real time, technology can be used to “decipher” facial expressions, analyze voice patterns, and track eye movements. With these, AI then produces music and other content designed to elicit specific emotional responses from users by mimicking human emotional intelligence. As such, AI-generated content can impact the users’ behavior and decision-making, including such important areas as how they vote, what they buy, what they eat, and even how they think of themselves. This raises concerns about the potential impact on free will and the moral implications of using AI to influence human emotions. The exact mechanism by which AI-generated content manipulates human behavior is not yet fully understood, but research[41] has identified several ways in which AI can cause emotional harm, create a false sense of intimacy, encourage attachment or addiction, objectify or commodify the human body, or cause social and/or sexual isolation. AI-generated content can also be used for more intrusive and effective nudging - emotionally altering or coercing human behavior toward certain products and services.
Deepfake scenarios typically involve three parties: a creator who produces the fake, a target who is misrepresented in it, and an audience who is affected by it. However, deepfakes can also be disturbing when the target and audience are the same, such as when individuals see deepfakes of their own past actions. These fabrications can disrupt or alter personal memories, known as Panoptic Gaslighting.[42]
Concerns about the use of AI to manipulate people's perceptions have sparked policy discussions about following Chile's path[43] and introducing neurorights to better protect citizens' cognitive liberty and privacy.[41]
Model Collapse and Reinforcement of Biases
The proliferation of synthetic content also runs counter to the interests of generative AI providers themselves. The development can have an irreparable effect on the quality of the output of AI models, in particular through a phenomenon known as model collapse.[44]
Model collapse refers to the degeneration that occurs in generation of output of Large Language Models when they are trained on polluted or synthetic data.[45] Without diving too much into the statistical details: instead of new human or real data, the AI systems are fed with previously AI-generated synthetic data, a kind of rumination of patterns learned by previous models.
This leads to a misinterpretation of reality by the models as they reinforce their own beliefs based on the synthetic data on which they were trained[46] with far-reaching consequences that affect the quality, reliability, and fairness of AI-generated content.
This may partly explain the self-interest of LLM providers behind their voluntary commitment[47] to watermark their output.
Devaluing Original Content and Market Distortion
As AI's ability to mimic human creativity grows,[48] it risks devaluing human artistic expression and sparking intense disputes over originality, ownership, and fair use. Authors John Grisham, Jonathan Franzen, and Elin Hilderbrand's copyright infringement lawsuit against OpenAI underscores this issue. They allege that OpenAI's ChatGPT, trained on their books, could create “derivative works” that could distort the market for the authors' original works.[49] Similarly, The New York Times has sued OpenAI, accusing the company of illegally using its articles to generate AI content.[50]
Tools such as Midjourney and Stable Diffusion, use extensive art and text databases to produce content that directly competes with human creators in the market. Karla Ortiz, a celebrated illustrator for Marvel movies and others, highlighted this in her testimony[51] before the U.S. Senate, discussing the unauthorized use of artists' work to train AI without consent, credit, or compensation, and emphasizing its impact on market dynamics. The sale of AI-generated artwork at significant prices has shaken the art world.[52]
Regulatory Requirements and Platform Policies
This troubling selection of developments, coupled with the industry's failure to effectively self-regulate, has led many countries to advocate for government intervention. Many jurisdictions around the world, as well as online platforms, have either proposed or implemented regulations aimed at requiring the disclosure of synthetic content. The goal is twofold: to promote transparency in content creation and to protect individuals from misinformation and misinterpretation.
Platforms like Facebook[53] and Instagram are enforcing policies that require informative labels for AI-generated content. Meta has announced[54] an expanded policy to label AI-generated images. TikTok[55] similarly requires clear labels such as "synthetic," "fake," "not real," or "altered" for synthetic media, and has launched a tagging tool for AI-generated content.
United States
In the US, the Schatz-Kennedy AI Labeling Bill[56] proposes clear and conspicuous disclosure for AI-generated content, aiming to ensure that “people are aware and aren’t fooled or scammed.”[57]
The White House Executive Order on the Safe, Secure, and Trustworthy Development and Use of Artificial Intelligence[58] also emphasizes the need to improve the identification and labeling of AI-generated content for government authenticity, including methods such as watermarking and synthetic content detection.[59]
European Union
In the EU, the Digital Services Act[60] requires very large online platforms to outline systemic risks related to societal harms such as the spread of illegal content, disinformation, cyberbullying, and their impact on fundamental rights and mental health. As a potential mitigation measure for identified risks, “Companies must take measures …, such as adjusting algorithms and implementing content labeling”.[61]
Article 35.1 (k) of the DSA requires that “a generated or manipulated image, audio or video that appreciably resembles existing persons, objects, places or other entities or events and falsely appears to a person to be authentic or truthful is distinguishable through prominent markings when presented on their online interface.” This could be realized through “standardized visual or audio marks, clearly identifiable and unambiguous for the average recipient of the service, and should be adapted to the nature of the individual service’s online interface.”
In the latest version[62] of the EU AI Act, as agreed on 2 February 2024 and published by the European Parliament - which may still be subject to change during the ongoing finalization of the text - Article 52(1a) of the EU AI Act requires "Providers of AI systems, including GPAI systems, that generate synthetic audio, image, video or text content shall ensure that the outputs of the AI system are marked in a machine-readable format and are recognisable as artificially generated or manipulated.”
Article 52 (3) of the EU AI Act requires deployers of an AI system that generates or manipulates image, audio or video content constituting a deep fake, to disclose that the content has been artificially generated or manipulated.
China
China's Interim Administrative Measures for Generative Artificial Intelligence Services ("GenAI Regulation") requires service providers to label such content as images, videos, and other content generated by AI in accordance with the provisions of the Practical Guidelines for Cybersecurity Standards - Method for Tagging Content in Generative Artificial Intelligence Services.[63]
A Technical Exploration of disclosure methods
Intersection with Media Literacy and AI Transparency
While our report focuses on disclosing whether content is generated by AI systems, it's important to acknowledge the broader context in which content disclosure is discussed. The concept of content disclosure has long been the subject of discourse in the fields of media literacy and platform regulation, where various methods have been considered. These include disclosure of data provenance, information about rights, consent in data collection, or distinguishing content as advertising or product placement.
Our topic is a subset of the broader topic of Meaningful AI transparency. AI transparency includes a wide range of dimensions, including providing comprehensive information to facilitate informed decision-making, ensure accountability, uphold fairness, and trace the chain of responsibility. It includes considerations such as data provenance and environmental and societal impacts.However, our report deliberately focuses on one specific aspect: disclosure of the synthetic nature of content.
Taxonomy of Disclosure Techniques
In the evolving field of synthetic content disclosure, traditional methods such as watermarking and labeling are blurring, with no fixed definitions. Our taxonomy, which focuses on governance and method suitability, distinguishes between human-facing, perceptible methods (visible or audible) and machine-readable, human imperceptible methods.
Human-facing disclosure methods, such as visible and audible disclosures, are designed to engage human audiences by being seen or heard. From a governance perspective, their suitability lies in their potential ability to directly and meaningfully inform and engage the public about the synthetic nature of the content. They serve as a transparency mechanism, allowing audiences to immediately recognize the involvement of AI. This direct engagement is critical in environments where public awareness and ethical considerations of AI-generated content are a priority.
In contrast, machine-readable methods are designed for machine recognition and interpretation. These methods play a key role in governance for the technical management of synthetic content, focusing on controlled tracking, distribution, rights management, and digital asset integrity rather than public engagement.
From a governance perspective, the decision to make this specific distinction between these categories helps in selecting the appropriate method based on governance objectives, whether it's human awareness or technical control and distribution.
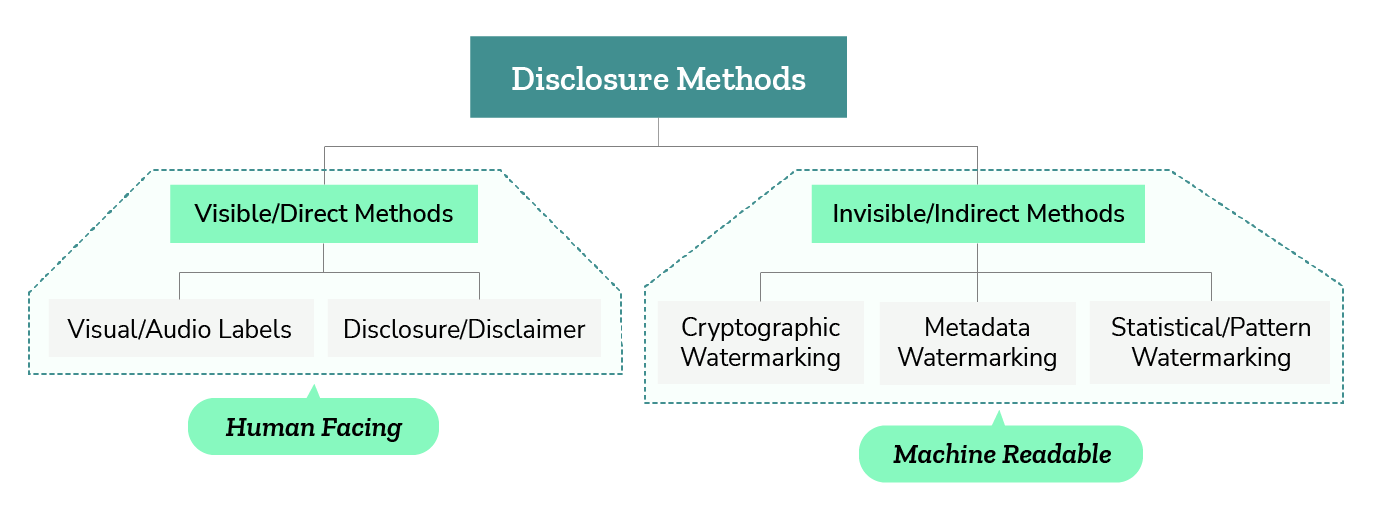
Image 5: Taxonomy of disclosure methods for synthetic content from a governance perspective
Human-facing Methods
Human-facing methods are those disclosure formats that present information about content in such a direct way that the user can perceive it – readable or audible – with their natural senses, without the need for detection software or other forms of machine-readable assistance. When one reads visible cues or annotations on content, or hears audio with insertions that remind them of information about the sound, or even reading through descriptions and disclaimers appearing in different formats in content, then a human-facing method of disclosure has been used. We note that these serve multiple purposes; including informing users as to whether or not a content has been created or altered by the use of AI. Further, they serve not just as a means of disclosure, but also to provide additional transparency to users and consumers of content. Methods of direct disclosure include content labeling, contextual annotation, visual or audible forms of watermarking, and disclaimers.
Visual/Audio Labels - Disclaimers
The concept of leaving an artist's signature on a physical work of art is a good example of visual labeling. Similarly, some digital content includes visual elements or annotations, disclaimers, tags, or nutrition labels that inform the user of the source or other important information about the content. Visual labels are “verbal or iconographic items”[64] (also) often used to disclose whether the content has been generated or manipulated by AI. It is a commonly proposed approach to alert users on social media or other information gathering sites that content has been synthetically generated - helping to quell the perceived negative impact that misinformation could cause.[65]
Human-facing labels could also take the form of audible content - audio/voice labels, that can be heard by human ears. Sound patches heard while listening to an audio content could sometimes contain information about the sound itself - a piece of music, or other type of sound. Imagine listening blindly to a piece of music or even watching a video with pauses containing sound patches - carrying information about the content; or radio jingles ending with patches like “This message was brought to you by”, or “I am a chatbot” when picking up a phone call. We note that there is not much research on the use of audible labels when compared to inaudible watermarks on audio content.
Like visible labels, audio/voice labels help identify whether a sound or voice has been generated or manipulated by AI. They also serve a variety of other purposes, including branding, copyright protection, content identification, and tracking. Consisting of cues or short clips, they can be placed anywhere in time-based content such as musical pieces or audio broadcasts, to help listeners distinguish between real and synthetic audio, and prevent the spread of misinformation or deception.
Whether automatically applied by a social media platform[66] or software[67], or subsequently attached to content by fact-checkers and journalists, visual and audio labels are directly consumable by the users and, as such, could stand as a frontline defense against misinformation or the spread of fake news.
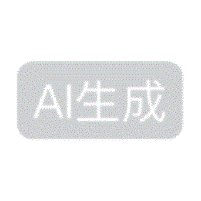
For instance, to comply with the new legal requirements in China, Douyin, TikTok's Chinese sister app, has implemented a platform policy requiring visual disclosure of AI-generated content with this icon.
Simple photo editing apps and software like Adobe Photoshop can be used to edit images and add visual labels. Audio labels could be easily recorded over existing audio or even video content.
On the other hand, visual/audio labels are also very easy to remove or alter. Sometimes, the process of tampering can be so intense that it also affects the original value of the content. Aesthetically speaking, human-facing labels - visual/audio, can be intrusive. Especially in the case of images, labels can extend into some visible/audible details of the content, covering some of its important information or aspects. This is especially true if they are designed to be noticed, which is the intention behind the disclosure. Since they (usually) already affect the aesthetic value of the content, the manipulation of audio labels can further impact the original value in many ways - for example, by leaving further audible dents or unexpected pauses in the content itself.
Sometimes, labels can be misunderstood due to contextual differences or language misunderstandings. A study conducted in the US, China, Mexico, Brazil and India, found that “the labels “deepfake” and “manipulated” were most associated with misleading content, whether AI created it or not.”[68] Moreover, with visual labels, as with Creative Commons labels, there is no guarantee of truth. Just as a person can use a public domain CC label to distribute another rights holder's copyrighted material,[69] human-generated content can be labeled as AI-generated and vice versa, failing, for example, to counteract the devaluation of original content. Labels can introduce bias against content, where terms like “AI-generated” can be misconstrued to imply distrust, even though not all AI-generated content is inherently untrustworthy.
Thus, even as they serve their primary purpose of disclosing the source of content or preventing potential misinformation, visual labels in AI-generated content are challenged by their aesthetic impact, susceptibility to manipulation, and potential bias.
Textual Descriptions and Disclaimers
Written information, whether in the form of captions, tags, or other forms of accompanying visible information, is often used to inform users about how the content was created or to warn users about the potential for harm of consuming that content.
This helpful guide[70] from “First Draft”, while focused on media literacy, offers an equally comprehensive overview of labeling methods that can be used to “simply” disclose the nature of the AI-generated content, ranging from pre-rolls, interstitials and bylines to profile information or annotations. Interruptions also serve as descriptive labels. These are like pop-ups in the course of the content, and are primarily used in video. For example, informing viewers that the following scenes in a video are synthetic, or periodically inserting this into a piece of content.
Description labels can be placed anywhere in the content. They can be pre-rolls - placed at the beginning of the content (in the case of videos) with descriptions of a content before the actual video starts, they can also be interruptions in the middle or in the course of a video, or such descriptive labels can be placed at the end of the content. The disadvantage - similar to audio labels - is the likelihood that some users will not watch the video or consume the content to the end before reacting to it or assigning any value to it. Another disadvantage is the ease with which they can be removed or simply clipped off (edited).[71] For descriptions that are just titles for content, a simple detachment could separate the description from the content - for example by downloading the video from the platform. Inaccurate descriptions can be even more damaging than not having them at all - they exacerbate the impact of misinformation. Such inaccuracies can result from contextual misunderstandings, language or even grammatical errors. Another downside is that these descriptions interrupt the flow of the content and can disrupt the aesthetic value of the content - as is the case with visible methods of disclosure. In the case of video, this is even worse.
Therefore, while descriptive labels are a flexible way to provide additional information about content, they have disadvantages, such as the risk that they will be removed or edited, and the possibility that errors will exacerbate the impact of misinformation. In addition, disclaimers are better suited to providing longer and more specific information, such as the source of the media or copyright terms, while visual labels, especially simple and popular icons, may be better suited if they are intended "only" to inform about the synthetic nature of the content.
The table below provides a comparison of the benefits and challenges of human-facing disclosure methods.
Pros & Cons - Human-facing Methods
Visual Labels |
|
---|---|
Pros |
Cons |
|
|
Audio Labels |
|
---|---|
Pros |
Cons |
|
|
Descriptions and Disclaimers |
|
---|---|
Pros |
Cons |
|
|
Machine- Readable (watermarking) Methods
Some customers are surprised to find out that the banknotes they brought to the bank are not authentic. At first glance, the signs on both the genuine and fake banknotes are the same, but banknotes have invisible watermarks - hidden pieces of information for authentication purposes. These are only readable through special detection devices or software. Similarly, content can be watermarked. This is one of the most commonly proposed[72] methods of effectively disclosing the presence or absence of synthetic content, and involves embedding a marker or a pattern (in the case of audio files) to identify an attribute of a particular content. Also known as digital watermarking, it is “the process of embedding information into digital multimedia content such that the information (which is called the watermark) can later be extracted or detected for a variety of purposes, including copy prevention and control.”[73] This could be pieces of data impressed on the content and made machine-detectable - as opposed to labels, which are easily and publicly identifiable. There is no consistent usage of the word watermark, some would also use watermark for visible marks. In our taxonomy, visible watermarks would be classified under Visual Labels, since the distinguishing feature would be human recognizability. Some authors have argued that watermarking is technically more suitable for non-textual synthetic content such as images, audio and video.[74] However, it is also possible to embed watermarks in certain AI-generated texts. In the following we introduce some of the most common non-visible watermarking techniques.
Metadata watermarking
This is another non-perceptual method, but unlike previous watermarking methods, it embeds information in the metadata of a digital file rather than in the content itself. Embedded information can include a wide range of information, such as author information, timestamps, and even details about the editing history and specific creation details of the file, such as the software used or the specific camera settings. It could also include an indication of the nature of the content as AI-generated. The primary purpose of using metadata for watermarking is to provide authentication information without altering the perceptual quality of the content, preserving its original visual or auditory characteristics. In many cases of watermarking, this information is intentionally added either at the time of or after the creation of the content.
However, it's important to note that the effectiveness of metadata watermarking can be limited. For example, many online platforms and file sharing methods can strip metadata from files, potentially removing the watermark. In addition, the ease of modifying or removing metadata compared to more integrated watermarking methods could be a potential downside. Large amounts of metadata can also increase file size, but this issue is not significant in the context of embedding information about the synthetic nature of the AI as the only information.
Frequency component watermarking
This pattern-based technique involves embedding a watermark in the frequency domain of a digital signal. It can be applied to various media, including audio, video, and images. The watermark is typically inserted in a way that minimizes perceptibility while ensuring robustness against manipulation. The process often involves decomposing the content into different frequency parts, and the watermark can be inserted into the low frequency bands that are less sensitive to attack and alteration.[75] The insertion scheme may include steps such as binarization of the watermark, use of error correction codes for better detection, and careful selection of insertion positions to ensure invisibility to the human eye. Because frequency-component watermarking works by altering pixel values to add additional information, this very modality, if done carelessly, can compromise the quality of the original content if the alteration is done in a way that is perceptually visible, especially if the watermark is overlaid as a logo or text. While the undetectable nature of this watermark makes it robust against certain manipulations, it can be difficult to embed and can affect aspects of the content, such as file size. Despite its complexity, it is still susceptible to certain levels of manipulation.
Cryptographic Watermarking
With cryptographic watermarking, information - such as signature - is embedded in programs or digital objects such as images, video or audio clips. In simple terms, cryptographic methods are like inserting locks that can only be detected, removed or changed with the key - an encryption/decryption process. Often referred to as a mark, secret information is encoded into the cryptographic functions (or circuits) of a content - to create an identity based on the presence of its existence without the ease of removal. Both marking and detection keys are used, in addition to detection and removal techniques.
While cryptographic watermarks are not particularly effective for text-based generative AI content, they do find their strength in binary file formats such as images, video, and audio files. The problem with text-based content is its vulnerability; cryptographic watermarks can be easily lost or altered in simple processes such as copying and pasting text because they are often embedded in formatting or metadata that doesn't transfer. In contrast, binary files provide a more robust medium for these watermarks. They can be seamlessly integrated into the data, creating a persistent identity that is much more resistant to easy removal or tampering.
Earlier this year, Meta introduced AudioSeal as "the first audio watermarking technique designed specifically for localized detection of AI-generated speech".
Google's SynthID is another example of encrypting and decrypting watermarks on content such as images, videos or even audio files. Users can easily add digital signatures to their own AI-generated photos or music. Although invisible to the normal human eye, such watermarks can be recognized by detectors for identification purposes.
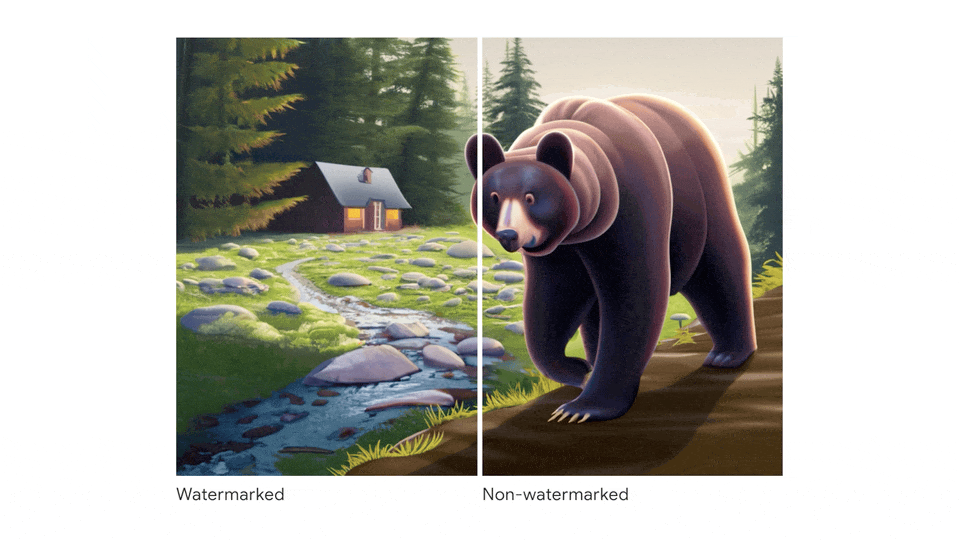
Image 6: Screenshot showing an invisibly watermarked image (left part) from Google DeepMind.
The Coalition for Content Provenance and Authenticity, supported by Adobe, Microsoft, Google and others, has developed the C2PA standard, which combines cryptographic watermarking and metadata embedding. It enables the insertion of a watermark into digital content, allowing cryptographically verifiable information to be stored and accessed to validate the origin and authenticity of the content.[76]
One of the major advantages of cryptographic watermarks is that - depending on the implementation - they can be difficult or nearly impossible to erase without damaging the content. And since they are invisible and undetectable by normal sight or sound, bad actors are less likely to even notice their presence on the content, thus reducing the likelihood of attempts to tamper with the content. Furthermore, they have minimal to no impact on the appearance or sound structure of the content. As a result, they preserve the original value of the content in which they are embedded.
On the other hand, it comes with disadvantages such as the fact that this method does not readily serve the primary purpose of public disclosure - by providing users with information about the origin of the content in which they are embedded. Users are not able to easily detect the watermark, as it requires special methods and detection programs to identify what is synthetic and what is not. Thus, they can still be deceived or manipulated into attaching more value to a piece of content than they necessarily should.
Statistical Watermarking
Also designed to be imperceptible to the normal human eye or ear, statistical watermarking is used to insert information into statistical patterns of the data structure of content - be it images, text, audio or video. This typically involves changing certain values of the content - such as pixels, color frames, sound components, etc. - without affecting the value of the content. Such changes are imperceptible to the human ear, but are machine-readable. Computer scientist Scott Aaronson is building such a tool for statistical watermarking for OpenAI. He explains[77] in his blog that:
GPT processes input and output as sequences of tokens, including words, punctuation, and word fragments, with a total of about 100,000 tokens. It predicts the next token based on the sequence of previous tokens, using a probability distribution generated by a neural network. This selection is influenced by a "temperature" setting, which introduces randomness. With a non-zero temperature, different outputs can result from the same prompt. For statistical watermarking, GPT switches from random to pseudo random token selection using a cryptographic function (key) known only to OpenAI. This change being usually undetectable to users unless they can distinguish between pseudorandom and truly random choices.[78]
Statistical watermarks are known to survive content manipulation. Certain forms of attacks do not completely change the watermark.[79] As an advantage, this makes statistical pattern watermarks robust, secure and reliable. Its imperceptibility forms the first line of security as it requires special methods to be noticed in a content. On the other hand, as with other forms of invisible disclosures, the imperceptibility becomes a disadvantage. Users looking at a watermarked image on social media platforms cannot instantly tell whether it is AI-Generated or not. This method is also very complex as it requires complicated algorithms to embed or decode. Although very robust and secure, it is not impossible to manipulate statistical patterns in a way that deceives the detector or decoder.
The Relevance of Detection Mechanism for Machine-Readable Methods
Since machine-readable methods lack the ease of readability of human-readable methods, robust detection techniques such as classifiers and content blocking structures are critical.
This study from GRIP demonstrates the effective detection of synthetic images, even after common post-processing operations, contributing to the validation of synthetic western blot images[80] using forensic techniques.
Furthermore, machine learning algorithms - especially when trained on a variety of datasets - help to create models that can recognize subtle patterns indicative of AI-Generated content.[81]
While detection methods are essential to give sense to machine-readable disclosure, more robust methods need to be developed. Detection tools/classifiers can be unreliable and inaccurate, regularly over- or under-detecting. For example, detection tools can be biased against non-native English speakers, whose submissions are more likely to be flagged as AI-generated, according to one study.[82] OpenAI launched its detection tool in January 2023,[83] but it was taken down in June with a statement due to its surprisingly low accuracy rate.[84]
The table below provides a comparison of the benefits and challenges of machine-readable disclosure methods.
Pros & Cons - Machine-readable Methods
Cryptographic Watermarking |
|
---|---|
Pros |
Cons |
|
|
Frequency Watermarking |
|
---|---|
Pros |
Cons |
|
|
Statistical Watermarking |
|
---|---|
Pros |
Cons |
|
|
Metadata Watermarking |
|
---|---|
Pros |
Cons |
|
|
Fitness Check
In the realm of legislative negotiation and reform, there is often a disconnect between the actual effectiveness of proposed regulations and governance strategies. In the midst of the complicated legislative process, it is imperative to assess whether regulatory actions are effectively addressing the issues they aim to address. Although it is widely recognized that the effectiveness of legislation is a key indicator of its quality, there remains a lack of agreement on what constitutes an effective law or on the methods to ensure its effectiveness. This concept often remains theoretical, embodying a goal or intention rather than being implemented as a tangible principle.[85]
De Benedetto highlights the challenge of making laws work efficiently, noting that "effective law raises the question of how it is possible to make rules work well.[86] Karen Yeung emphasizes that for technology regulation to be effective, it must not only mitigate the direct and indirect negative effects of technological progress, but also steer its development in a positive direction.[87] Following Xanthaki, “A good law is simply one that is capable of contributing to the production of the desired regulatory results.” Effectiveness becomes a fundamental aspect of the principles that determine the quality of legislation, reflecting the relationship between a law's intent and its effect, showing how well it influences the targeted behaviors and attitudes as envisioned by the legislator.[88] Essentially, effectiveness measures how well an intervention "gets the job done" and becomes a primary indicator of the quality of the intervention.
In the absence of clear standards, we can rely on more general criteria to evaluate the success of technology governance measures. The OECD provides a comprehensive framework for evaluating the impact (including merit, value or importance) and efficiency of various interventions such as policies, strategies, programs, projects or activities.[89] An intervention may take the form of a regulatory requirement,[90] such as a mandate for watermarking or labeling.
These are the evaluation criteria and key questions from the OECD framework:
Relevance | Does the intervention address the right issues, meet the needs of beneficiaries, and adapt to changing circumstances? |
---|---|
Coherence | How well does the intervention fit in with other initiatives? |
Effectiveness | Does the intervention achieve its objectives and produce the expected results, including any differential impact on different groups? |
Efficiency | Are resources used optimally to achieve results in a cost-effective and timely manner? |
Impact | What significant changes, positive or negative, intended or unintended, does the intervention bring about? |
Sustainability | Are the benefits of the intervention durable and likely to continue? |
The core element of the fitness of interventions such as policies is the question of their effectiveness in terms of their potential to achieve the goal. The goal of an intervention is to prevent and, in the best case, to control the above-mentioned undesirable developments and harms.
Using the terminology of the European Commission, this section therefore provides a "fitness check"[91] to assess the potential impact of human-readable disclosure methods on the one hand and machine-readable methods on the other in addressing the identified harms.
Guided by the OECD's output-oriented evaluation criteria, we focused our Fitness Check on the core feature, the effectiveness of the intervention and, potentially, its sustainability. Our Fitness Check, which looks at human- and machine-readable disclosure methods, is a purely subjective tool that aims to link the theoretical intent of a regulation with its potential real-world impact. It is intended as an invitation to take a critical look at regulatory quality through the lens of effectiveness. It is not a judgment on the quality of the methods themselves; a technical method may be robust in itself but still fail to achieve the regulatory goal of reducing potential harm.
Fitness Check
Human-Facing Disclosure Methods
Effectiveness: The success of human-facing disclosure methods for AI-generated content, similar to health symbols on food packaging, depends on consumer understanding and motivation.
Visible labels may also fail to prevent harm that has already occurred, especially in the case of non-consensual deepfakes, or to effectively address perceptual harm, making them less effective when they are most needed. Already objectified, devalued, and in a defensive position, potential victims will already experience negative emotions and helplessness.
The appropriateness of methods depends on the context. Even if content creators have a vested interest in labeling their content, they may be reluctant to use them if they compromise aesthetics. Moreover, the intrinsic compliance potential of mandatory disclosure is questionable, as malicious actors can and will choose not to label content.
In addition, human-facing methods are vulnerable to manipulation and removal.
Sustainability: Mandating specific measures when the state of the art is constantly changing can lead to unsustainable practices. Challenges such as mislabeling, whether malicious or unintentional, can make it difficult to establish clear and consistent labeling guidelines. Enforcing human-faced methods can be resource-intensive and difficult to implement. And if Gartner's prediction that 60% of the data used to develop AI and analytics will be artificially generated by this year comes true, it's questionable how long labeling will remain a viable approach.
Solutions could create new problems: Human-facing methods can exacerbate public distrust and deepen societal divisions, and they don't address broader societal implications, such as the impact on work, education, and democracy. Visual labels can undermine trust in media and public discourse, fostering uncertainty and cynicism.
Emphasizing transparency not only as a prerequisite for effective governance and oversight, but also as the primary mitigation measure, places the onus on users as recipients of even more information.
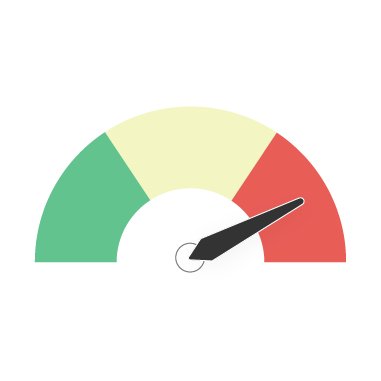
Overall Fitness: LOW
Effectiveness: Machine-readable watermarking techniques can be effective when implemented during content creation and distribution. The effectiveness of these methods against harms such as identity theft and other security vulnerabilities is enhanced by their relative security against tampering and removal by malicious actors.
However, the success of machine-readable disclosure methods depends heavily on the existence and quality of robust, unbiased and reliable detection mechanisms. Current watermarking schemes are not designed for detection. In cases where such mechanisms are missing, which is often the case, their effectiveness is significantly reduced.
Tech-solutionism: A purely technological approach, often referred to as tech-solutionism, may hinder the development of a holistic and balanced governance strategy to address the harms caused by AI-generated content and its large-scale distribution. Such a strategy should include not only technology, but also education and new ideas to address issues at multiple levels simultaneously. The focus on watermarking and detection tools diverts attention from broader systemic issues, such as the role of targeted political advertising in shaping public opinion.
Combining measures to achieve better results: For example, while watermarking can reduce the risk of accidentally training a model on AI-generated content, which can reinforce existing biases, the effectiveness of bias reduction is increased by using carefully curated, high-quality data and addressing the root causes of discrimination and bias.
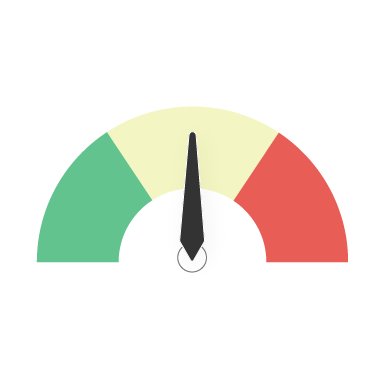
Overall Fitness: FAIR
In our fitness check, none of the methods mentioned is a silver bullet – none represents a complete solution or comprehensive remedy.
While transparency is essential for trustworthy and beneficial AI, and digital watermarking can be used as a “triage tool for harm reduction”[92], disclosing syntheticity can be part of the solution, but not a comprehensive remedy for the challenges posed by fake news and misinformation related to elections, or the distribution of child sexual abuse material, where the harm may have already been done with the perception of the content. Disclosure alone cannot be a substitute for a necessary holistic approach that requires a combination of technological, regulatory, and educational approaches to effectively address harm.
We agree that different regulations are effective in different ways.[93] Thus, meaningful disclosure requires a holistic approach[94] that combines technological, regulatory and, most importantly, educational measures. As one would notice on the table, certain methods are best for certain purposes – for instance, labels are good for social media platforms, where users can easily identify them. Machine-readable methods by themselves would not be fit for this purpose. So a community use of methods is recommended. Machine-readable disclosures coupled with making available detection systems and, informing or educating users on synthetic contents and the harms they bring, would serve as a more effective and sustainable strategy towards mitigating the discussed harms of synthetic content.
Overall, we emphasize the need for human-centered technology policymaking. By establishing venues for authentic public engagement, policymakers gain valuable perspectives on the real-world impact of technology policies on individuals. In addition to enhancing the legitimacy of policies, this inclusive and participatory methodology ensures that the many demands and concerns of society as a whole are taken into account, resulting in regulatory frameworks that are more responsive and effective.
It is also particularly important for effectiveness that the responsibility for embedding the measure lies with the right place: where the content is generated and distributed.
“Taking human rights seriously in a hyperconnected digital age will require that effective and legitimate governance mechanisms, instruments and institutions are in place to monitor and oversee the development, implementation and operation of our complex socio-technical systems.”
Quote from A study of the implications of advanced digital technologies (including AI systems) for the concept of responsibility within a human rights framework (2019)
Our Recommendations
The governance of AI infrastructures goes beyond tech policy and requires holistic approaches, ethical considerations and the development of frameworks and strategies to ensure transparency, security and robustness of systems, fairness, protection of informational self-determination and privacy, adaptability and accountability. In addition to effective legislation, this also includes the development of alternative beneficial and trustworthy AI Technologies that are transparent in such a way to allow for independent verification (auditing) and assurance (safeguarding through red-teaming, etc.) throughout their lifecycle. Tech policy needs innovation to keep pace with technological development. Our recommendations are intended to encourage multidisciplinary efforts and societal participation along this path together in order to enable desirable technological developments.
Detection & Disclosure Approaches
- Prioritizing machine-readable methods:
Depending on the context and platform, as well as the specific harm to be mitigated, efforts to disclose AI-generated content should determine which of the two types of disclosure (human- or machine-readable), or both in combination, are needed. In terms of method effectiveness, we recommend that priority be given to the development of standardized, machine-readable, robust, and tamper-resistant watermarking methods coupled with efficient detection systems. These methods, applied at the point of content creation and distribution (see above), are more effective than those that require direct human understanding.
Human-facing methods tend to shift responsibility to the end user, who may already be overwhelmed with too much information and too many choices. This would also reduce the burden of enforcement. - Slow AI: Law is only one pillar of technology governance. AI governance should put more emphasis on the development and implementation of technologies that are fair, safe and clean in their use. We advocate for the promotion and allocation of more R&D and funding for 'slow AI' solutions (derived from 'slow tech'[95]) that embed corporate social responsibility into technology. For example, labeling and detection systems for generated content could be tested for effectiveness before an AI system is rolled out, and a technology could only be brought to market after ensuring that potential pollution or harm has been addressed or avoided in the first place.
- Balancing Transparency with Privacy: Watermarking, such as extensive tracking of the entire editing process in Photoshop - including every step of the creator - can help determine the degree of artificiality in content. However, it pushes the boundaries unnecessarily, leading to surveillance of designers and content creators that threatens privacy. Unfortunately, it can also expose political activities that confidentially create content in vulnerable structures and situations. Therefore, it is critical to distinguish between meaningful transparency and expanding surveillance, eliminating safe spaces, or violating artistic freedom in the name of data integrity by preventing technology providers from implementing surveillance methods that are excessive and interfere with citizens' rights.
- Ensuring that unbiased detection mechanisms are widely available and standardized: Adopting and ensuring the widespread availability of secure and unbiased detection technologies is essential. A global standard for detection methods is also recommended. This would not only help streamline enforcement, but also provide clear, practical guidance for technology developers.
- Exploring Open Source Watermarking: Closely related to accessibility of methods, it is recommended that the feasibility of open source watermarking and detection methods be explored. This could lead to more innovative and accessible ways of ensuring the authenticity of content, while addressing the risk of misuse by malicious actors.
Tech Policy Strategies and Innovation
- Holistic and balanced strategy against synthetic content harm: While we advocate greater efforts to use technology to mitigate harm, techno-solutionism is not the goal. While useful in parts, none of the disclosure methods will be sufficient to eliminate the harms outlined above. Given the limited impact of current disclosure methods, innovative and more specific strategies are needed to address the challenges of synthetic content. For example, Watermarking is not entirely effective in mitigating the risk of fake news and misinformation manipulating citizens in elections. While watermarks can help distinguish between real and fake content, they are not infallible and can be manipulated or removed by bad actors, potentially leading to further spread of misinformation. A multi-faceted strategy to prevent both intended and unintended harm should include user education, legislative action, and specific Tech development to effectively counter the proliferation of harmful synthetic content.
- Voluntary requirements are not enough: Researchers[96] have noted that relying on voluntary self-disclosure may not fully serve the purpose, as bad actors may refuse to label or watermark, mislabel or even provide misleading labels or marks on content. Requirements should be mandatory.
In fact, the approach taken by the Digital Services Act (DSA) in Article 35.1(k) strikes a balance by requiring that information that resembles a real person, object or event, but is generated or manipulated by AI, be covered without prescribing specific solutions. This flexible framework allows for tailored responses to the unique challenges posed by AI-generated content. The DSA's requirement for large online platforms and search engines to implement reasonable, proportionate and effective mitigation measures, taking into account the specific systemic risks identified under Article 34, is a pragmatic strategy. Standards and best practices can assist developers in implementing these measures. - Assigning Responsibility for the Marking at the Point of Origin: Explicit, fake images of Taylor Swift posted on X were viewed 47 million times, upsetting her fans and sparking renewed calls from lawmakers to better protect women before the account was suspended.[97] To prevent harm, it is necessary to stop the dissemination of harmful AI-generated content.
To avoid the distribution and downstream use of harmful synthetic content, the responsibility for implementing these methods should lie primarily with the entity that creates the AI-generated content (point of generation/backend). Where content creation and distribution are separate, the platform disseminating the content (point of distribution) should also be responsible. As OpenAI and other GenAI providers move toward becoming platforms, similar to VLOPs, regulators should be quick to recognize the new developments. - Re-imagining Regulatory Sandboxes: Technology is created in iterations, constantly evolving and adapting, yet the law that governs it, such as watermarking and labeling of synthetic content, is expected to be flawless and future-proof from the outset - an unrealistic expectation. Ineffective regulations, once introduced, will block other regulatory approaches for many years. It is very important to test their feasibility before implementation. Regulatory sandboxes are being experimented with in many countries, particularly in the fintech space, and represent an innovative approach to legislation and enforcement. Their primary focus has been on accelerating the time to market for new technologies and helping providers meet regulatory requirements.[98] However, this approach overlooks a critical aspect: AI is not just a technological tool, but a socio-ecological system.[99] The existing model of regulatory sandboxes doesn't adequately involve citizens in testing methodologies, such as deepfake disclosure requirements, and thus misses an opportunity for a deeper understanding of social and environmental impacts, including the effectiveness of disclosure methods for synthetic content.
With the introduction of regulatory sandboxes under the AI Act, why not use them to experiment with new governance concepts and rigorously test existing regulations, particularly in monitoring and enforcement, and periodically update the law? Such an evaluation cycle would essentially require an environment where changing the law doesn't always trigger massive lobbying for deregulation, a regulatory safe space. - Exploring the use of Legal Tech to enforce Tech Policies: While technology is increasingly used to enforce laws against citizens in areas such as policing, border control, 'emotion' recognition, DRM systems, upload filters and surveillance, its use to protect citizens is rare and limited to a few legal tech tools to enforce consumer rights and small initiatives for privacy enhancing technologies such as cookie and in-browser tracking blockers.
Legal tech has the potential to play a significant role in this area, particularly in enforcing policies that require the disclosure of AI-generated content and enhancing detection mechanisms to identify such content. The potential of this technology to protect citizens is underutilized and underexplored. - Developing meaningful success metrics and indicators for the effectiveness of regulation to add measurability and evidence-based methods to Tech Policy.
- User awareness and education: We strongly emphasize the need for user education about synthetic content. Many users do not understand how to identify and understand the implications of AI-generated content, even when it is labeled or watermarked. Education is key to building citizen resilience and complements, rather than replaces, effective regulation.
Methodology
In order to illustrate the main objective of our research - the effectiveness of disclosure methods for synthetic content - we conducted desk research for a comparative analysis and review of different disclosure methods and watermarking techniques, as well as legislation requiring disclosure in the realm of synthetic content production. We synthesize insights from existing research papers and reports, searching also for trends, make inferences about the effectiveness of various watermarking techniques, and detect patterns.
Despite the robustness of our methodology, it's important to consider its inherent limitations. Scarcity of data due to the fast movements in the field or insufficient prior research in certain areas hinders exhaustive analysis. However, these limitations provide valuable guidance for future research inquiry in the advancing field of synthetic content disclosure and AI Governance.
Acknowledgement
We would like to thank the following reviewers for their invaluable time and insights. In alphabetical order, we thank Jesse Mc Crosky, Solana Larsen, Kasia Odrozek, Lucy Purdon, Nazneen Rajani, Becca Ricks, Raj Singh, Sebastian Stober, and D'Andre Walker. We also sincerely thank Claire Pershan, Mozilla's EU Advocacy Lead, for her deep tech policy expertise, unwavering commitment, and innovative efforts to connect our AI transparency research with Mozilla advocacy initiatives. In addition, we would like to thank Arafath Ibrahim for the outstanding artwork he provided for our report.