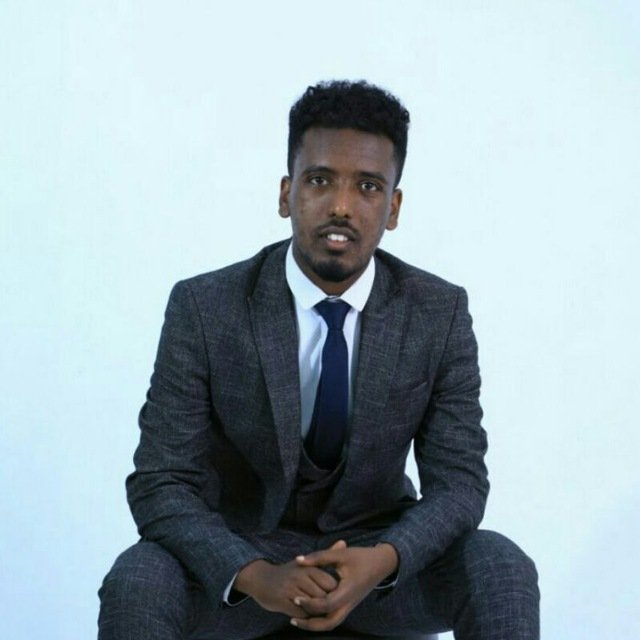
A feature of the winning project for Mozilla’s Common Voice, Africa model sprint, ‘Our Voice’ competition. In conversation with Yonas Chanie
From turning bedroom lights dimmer to playing music, to setting reminders — interconnected voice assistant technologies are the ever-looming invited guests in our homes today. But beyond these simple tasks, voice recognition technology is also making a difference in accessing life-saving information and mitigating widespread COVID-19 infections in Rwanda.
The Mbaza chatbot was a pivotal tool for locals in Rwanda in accessing information about the COVID-19 pandemic. Accessible in the local language, Kinyarwanda, the bot provided crucial information about where to get COVID-19 vaccines, lockdown restrictions, and any other relevant information to stay healthy. With an access reach of over two million users, Mbaza is inspiring budding voice technologists to create well-performing speech-to-text models for African languages.
Engineering and Artificial Intelligence graduate students from Carnegie Mellon University Africa: Yonas Chanie, Moayad Elamin, and Paul Ewuzie are the winners of the Common Voice African Languages STT Model Sprint. The competition incentivized voice technologists to design well performing models enhancing linguistic diversity and inclusion issues in the voice tech space.
The team worked together with Digital Transformation Center, which also supports Digital Umuganda (the project behind the Mbaza Chatbot), and utilized NVIDIA’s open-source NeMo toolkit to train a speech to text recognition model for Kinyarwanda.
So what does it take to use crowdsourced data from volunteer contributions to train a voice recognition model? The first approach was a noise extraction exercise, where he used the word rate technique which involves calculating the number of words per second and discarding audio samples that have a word rate greater than three seconds.
‘The data always had background voices and inconsistencies, and with a large dataset of around 2,000 hours and 57 gigabytes, these inconsistencies can heavily impact the performance of the model. For instance, how accurately a model translates an audio file into text with minimal errors,’ Chanie explains. They also removed other characters such as question marks to reduce inconsistencies.
The model was evaluated using the Word Error Rate metric and received an error rate of 17.5%, indicating good performance. Word Error Rates are used to measure speech-to-text accuracy of automatic speech recognition (ASR) systems. The lower the error rate, the better its performance.
Chanie hopes to advance the model and use it as a standard in working with other African languages such as Kiswahili and Luganda. ‘I am currently writing a paper on the work we did, and I hope it can provide a blueprint for future model designs,’ he says.