In the below post, Mimi Onuoha describes Ground Truth, a project in collaboration with Patrick Ball of the Human Rights Data Analysis Group which uses machine learning to infer the locations of undiscovered mass convict leasing-era graves in the US. By combining original research, art, and emerging computational techniques, the project foregrounds the lasting contemporary effects of the historical violence laced in US land.
Ground Truth is in part funded by MozFest. During the festival, Mimi Onuoha, Mikayle Green, Tami Ajeigibe, and Jack Sullivan will discuss the challenges surrounding the collection of the data necessary to create Ground Truth.
Ground Truth began with the discovery of a mass grave.
The grave in question was located in Sugar Land, Texas, the city I grew up in. In 2018, the town's local school district broke ground for a new school. In the process of digging, the team unearthed the bodies of 95 Black people. Local authorities soon determined that the remains were from people who had died between 1879 and 1909 as a result of the violent nineteenth-century practice of convict leasing.
Convict leasing, which saw its height during the period of Reconstruction but continued for decades after, took advantage of the loophole in the 13th Amendment that prohibited involuntary servitude for all except those convicted of crimes. In some Southern states, it became common practice to charge Black people with outsized punishments for petty "crimes." The state could then lease these folks’ labor to private planters or industrialists who paid minimal rates and were responsible for housing and feeding. The participating states profited from such arrangements, as they no longer had to pay to house convicts and often demanded up-front fees from those to whom they leased the convicts. Those who bought the convicts' labor profited as well: they had a cheap workforce who couldn't demand better conditions and whom they could, in some states, work to death and replace with impunity.
For the mostly Black people caught within the system, convict leasing served as a model of the type of racial domination we could be subject to at the hands of the state. This was the first time in U.S. history that many state penal systems held more Black prisoners than white, and it was also the first time that a capitalist desire for profit through industrialization and a coerced workforce was so nakedly tied to a white supremacist interest in racial domination at a state level. It would turn out to be a kind of blueprint. This is all the more noteworthy because of how removed (even nonexistent) convict leasing is today from the public imagination.
The folks in my hometown were no exception. Sugar Land is part of Fort Bend County, which is heralded as one of the most ethnically diverse counties in America. The fact that the city today has near equal proportions of the nation's four major ethnic groups (Asian, Black, Latino, and white residents) has made it something of a multicultural ideal. Though many residents are — like me — immigrants, others have lived in Sugar Land for generations. For those who took notice, the discovery of the "Sugar Land 95" caused a ripple of unease. How could our pleasant and innocuous town sit atop such a brutal past?
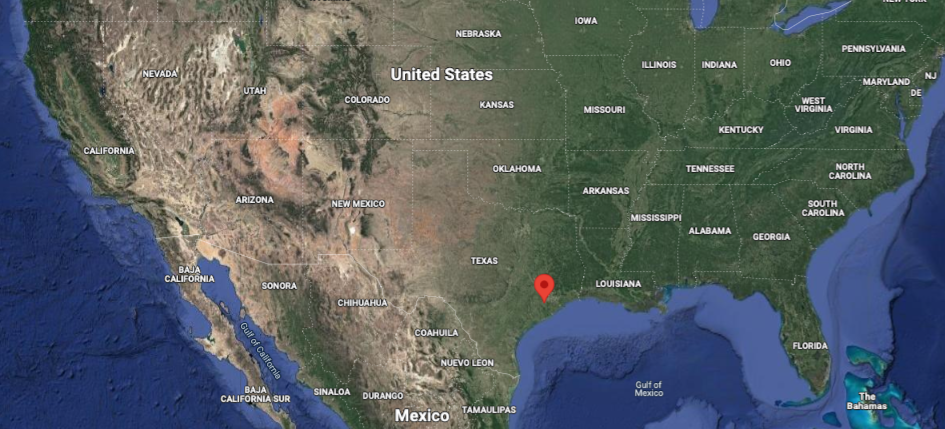
Finding patterns through evidence
In Ground Truth, I am interrogating the structures and practices that maintained the careful, collective unknowing of my hometown's history despite the steady presence of stories and evidence to the contrary. I am exploring why the bones of the Sugar Land 95 were the only proof allowed to cut through that veil of unknowing. And I am telling a story that positions the Sugar Land 95, and the grave they lay within, as what it truly is: national pattern rather than isolated case.
Since mid-2019, I have been working with the Human Rights Data Analysis Group (HRDAG) to build a machine learning model to estimate the likelihood that other sites in the United States contain convict-leasing-era mass burial graves like the one in Sugar Land. HRDAG and I have crossed paths around similar work in the past. While a data journalist at Quartz, in 2016 I wrote a story on the team's successful model that predicted which counties in Mexico were most likely to hold hidden graves full of desaparecidos, or missing people. The model that HRDAG published in collaboration with local partners is still used today by activists and families seeking to determine where the bodies of their loved ones might lie.
Though similar in structure to that work, Ground Truth contains notable differences. The ability of our machine learning model to predict similar sites in the United States relies upon the fidelity of the model's access to a trove of datasets which together can be used to extrapolate complex relationships of violence. Like all data, this information doesn't exist on its own. We have to collect and string together various artifacts to create it, a tedious task that requires digging through disparate sources—primary documents, historical archives, community and researcher databases, and oral accounts. We’ve made a significant headway on this work only due to the help of groups of Research Aides who have joined us at various points in the project.
Yet there’s much left to do. A model like this needs to be fed examples of places that we know with certainty have such graves so that it can detect other counties with similar attributes. But it also needs to know which areas do not have such graves in order for it to be able to register the differences between the two groups. What this means is that a large amount of our research is tied to the task of finding these negatives, these places where there are certifiably no convict leasing graves, a mission that’s harder than it seems. Most researchers are trained to focus on finding patterns through evidence that exists, the implication being that there’s no need to waste time on what isn’t there. Yet our model only will work if we pay just as much attention to the negatives as the positives.
The model that we ultimately create will function as a form of evidence that may even hint towards the possibility for long-needed collective reckonings. It will be mathematically and computationally sound, but there’s no denying that it carries within it countless impossibilities. There’s the impossibility of actually digging for remains in any of these areas. There’s also the impossibility of scientific methodology to attend to grief, pain, and accountability.
Highlighting both the past, and future
These impossibilities are tied to the larger question that lies at the heart of this project: why do we need machine learning to tell us things that the land we live in already bears witness to? For years before the official discovery of the Sugar Land 95 there have been people in Sugar Land who have insisted on a recognition of the area’s history. For anyone willing to look, the layers of violence that created the city lay in plain view: the streets I played on as a child are named for the plantation owners whose wealth made the town; the middle school I attended carries a name from the very Indigenous groups who were eradicated by white settlers; even the name ‘Sugar Land’ speaks to the notoriously violent sugarcane plantations that the area was known for. The violence might have been in plain sight, but there were structures of unknowability that kept this history out of view for newcomers to the area and out of bounds for longtime residents.
The fact that these structures are beginning to crumble says more about the present than the past. And the fact that machine learning can play a role in pushing past these walls speaks to what forms of knowledge are valued and seen as trustworthy today. In this project, we’re using machine learning to question the mindset that makes belief in the veracity of these technologies so satisfying. Thus, this project is research as art, model as artifact, and about what can cut through that which white supremacy cloaks. The impossibilities are not just the heart of the project; they are what give it its strength. For though Ground Truth focuses on zones that have held layers of violence for Black and brown people, such areas are also sites of potential, places where alternative ways of considering the land’s history and future can be realized.
Ground Truth has been made possible due to the support of Mozilla Foundation, Creative Capital, the Eyebeam Center for Arts and Technology and HRDAG and the many Research Aides who have contributed their time to the project: the 2021 group of MiKayla Green, Jack Sullivan, and Tamilore Ajeigbe; and the 2020 group of Gus Brocchini, Benazir Neree, Julia London, Brianna Turner, Samantha Gamble, Gabriella Gordon, Sandra Ojeaburu, Sterling Lee, Chioma Onuoha, Maisam Goreish, Ian Hayes, Edidi Adjagbodjou, Celine Ibrahim, Marie Ayiah, and Christiana Ofosu.
Mimi Ọnụọha is a Nigerian-American artist and researcher whose work highlights the social relationships and power dynamics behind data collection. Her multimedia practice uses print, code, installation and video to call attention to the ways in which those in the margins are differently abstracted, represented, and missed by sociotechnical systems.
MozFest is part art, tech and society convening, part maker festival, and the premiere gathering for activists in diverse global movements fighting for a more humane digital world. To learn more, visit www.mozillafestival.org.
Sign up for the MozFest newsletter here to stay up to date on the latest festival and internet health movement news.