“I agree” to the Terms-of-Services (ToS) is perhaps the most falsely given form of consent on the Internet. The recent Zoom ToS controversy and backlash drew public awareness to technology companies' power to inconspicuously change their terms so they can build new kinds of proprietary generative AI models. While emerging startups and technology companies rush to deploy ever more powerful models, misleading contractual agreements such as ToS, data governance, and content policies, are becoming more common — and more consequential.
But this flawed form of consent doesn’t have to be the status quo. New kinds of consent models that center trust, transparency, and human agency are emerging, especially in cases of risks and harms of AI. These mechanisms draw on interdisciplinary fields such as human-computer interaction, privacy, legal design, and feminist science and technology studies. A vision for an alternative user agreement and multi-stakeholder engagement framework is the centerpiece of the Terms-we-Serve-with (TwSw) initiative and a related upcoming academic article to be presented at the ACM conference on equity and access in algorithms, mechanisms, and optimization. Adopting the TwSw in practice results in:
- reparative human-centered user agreements
- user studies and user experience research
- an ontology of user-perceived AI failure modes
- contestability mechanisms that empower continuous AI monitoring grounded in an ontology
- mechanisms that enable the mediation of potential algorithmic harms, risks, and functionality failures when they emerge
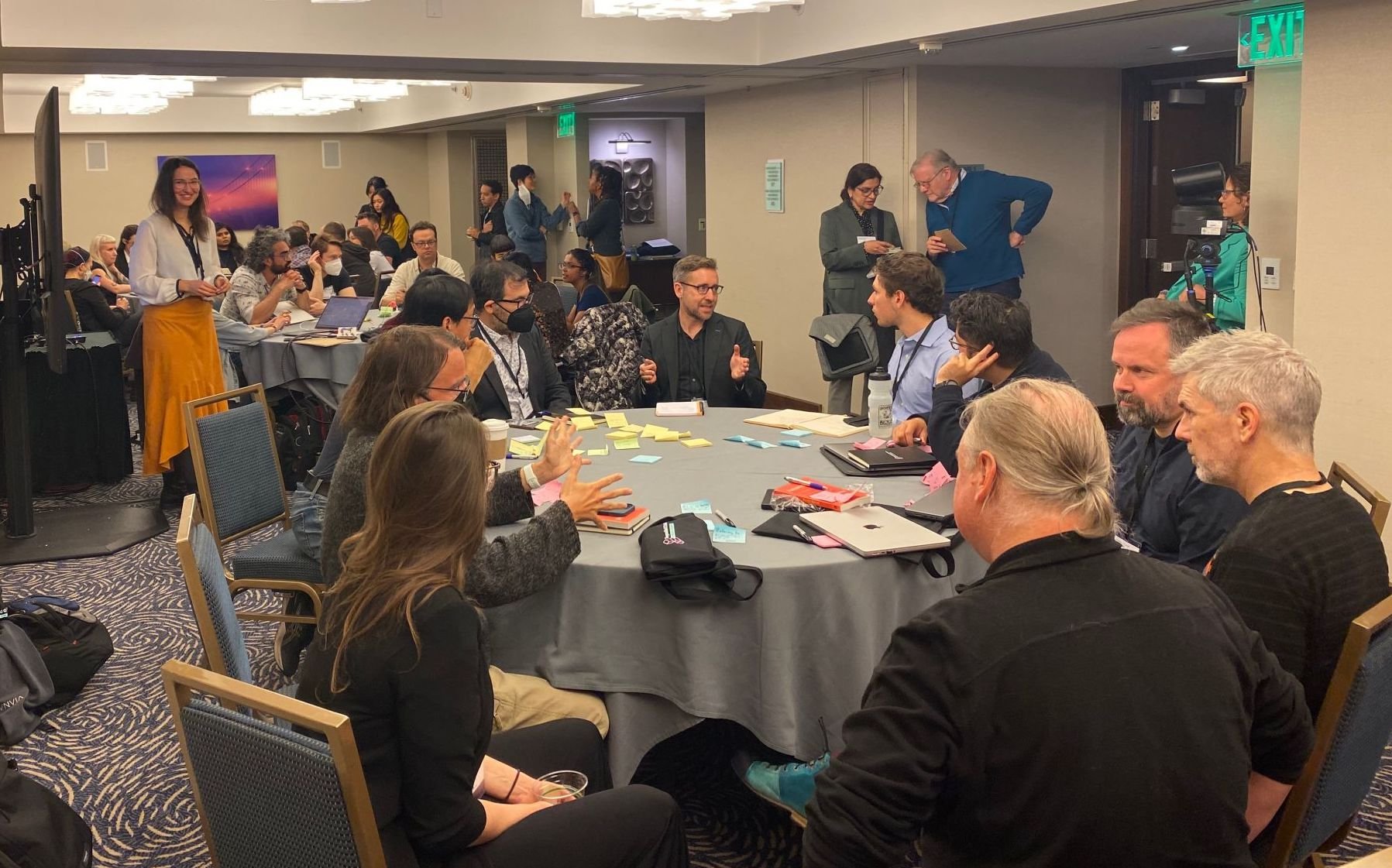
In this blog post, we lay out starting points for how rewriting clauses in a ToS agreement could enable a reparative approach to user agreements. Rooted in the call for algorithmic reparation by Jenny L. Davis, Apryl Williams, and Michael W. Yang, we define such a reparative approach as one that names, unmasks, and undoes allocative and representational harms as they materialize in sociotechnical form.
Human-centered contextual disclosure of data and AI governance
Drawing on our experience in using the TwSw framework in practice, we propose that user agreements and design interfaces need to contextually disclose and explain how a product or service uses algorithms, machine learning, or other kinds of automated decision-making or AI systems as well as their potential failure modes and downstream risks. This would include a disclosure of what data is collected from users and how it is used, for example, in the context of building or improving algorithmic models. Human-centered disclosure could also allow for AI companies to meaningfully respond to calls for critical AI literacy. For example, educators Maha Bali, Kathryn Conrad, and others have argued that there’s a need to improve user literacy of AI systems as well as their capacity to know when, where and why to use it, for what purpose, and, importantly, when NOT to use it.
User agreements and design interfaces need to contextually disclose and explain how a product or service uses algorithms, machine learning, or other kinds of automated decision-making.
~
In a recent template for voluntary corporate reporting on data governance, cybersecurity, and AI, Jordan Famularo, lays out disclosure prompts that companies can follow, including:
- Disclose a privacy and/or data protection policy that covers the organization's entire operations, including third parties. Specifically, each type of user information the organization collects; how is the data collected, how is it processed and with whom is it shared and for what purposes; the duration of time for which the organization retains user information; the process for responding to third-party requests (by both government and private parties) to share user information; and whether the organization conducts robust, systematic risk assessment for targeted advertising policies and practices.
- Disclose whether the organization has a cyber and/or information security team, including whether the organization has established an incident management plan that includes plans for disaster recovery and business continuity; disclosures of the impact of data breaches and security vulnerabilities; and description of policies and practices to secure customers' consumer health data and personal information.
- Disclose the organization's policy for AI governance, including the range of purposes for which algorithmic systems are used; how the organization takes action to eliminate racial, gender, and other biases in algorithms; and whether the organization conducts human rights due diligence and/or auditing to identify and mitigate the potential risks of algorithmic systems.
Contestability mechanisms and third party oversight for incidents reporting
Contestability is defined by academic scholars as the ability for people to challenge machine predictions. We expand that definition to encompass contestations along the life cycle of algorithmic systems including the data they rely on.
Systems designed for contestability could provide more meaningful transparency and human agency to their users, contributing to building trust-based relationships. Examples of contestability mechanisms are incident reporting systems, customer feedback forms, community forums, design choices that enable user feedback, for example, thumbs up or thumbs down options, or opportunities for feedback within the human-computer interface through which people engage with a technical system. There is a large body of interdisciplinary research that explores such mechanisms. Contestability interventions need to be made explicit through the user agreements encompassing AI products and services. This could then further legitimize their use and contribute to improved ability to conduct algorithmic audits. Furthermore, there’s a need for external oversight of data reported through such contestability mechanisms. Thus, technology companies could consider adding the following terms to their ToS agreements:
You should voice any perceived experiences of harms of this Service. You can do so through providing your feedback via our contestability mechanism. Feedback provided through our mechanism will be verified and reviewed by an independent external accountability forum ___.
We agree that any dispute or claim between you and [company] arising out of or relating to this Agreement or the Services will be resolved through arbitration or mediation.
Furthermore, limitations of liability clauses within ToS agreements should specifically address sociotechnical harms of algorithmic systems.
[THE COMPANY] AND ITS AFFILIATES AND EACH OF THEIR LICENSORS, AND SUPPLIERS WILL NOT BE LIABLE FOR ANY... INDIRECT, INCIDENTAL, SPECIAL, CONSEQUENTIAL, EXEMPLARY, OR PUNITIVE DAMAGES TO THE EXTENT THAT THERE IS NO DEMONSTRABLE HARM, EITHER INDIRECT OR DIRECT, SHOWN.
These changes in ToS agreements open the door for fundamental shifts in the relationship(s) governing how we engage with AI systems. They urge companies to move away from the fiction of consent in click-through contracts that nudge users to make tradeoffs they are not aware of.
Engagement and active co-design of user agreements
Innovation in user agreements could also point to interventions that empower new social relations and organizing structures that can function as collective guardrails in how technology is adopted. Building on the work of scholars who have urged regulators to consider how existing contracting regimes are dehumanizing and that technology can be predatory, our goal with the Terms-we-Serve-with is to point to the possibility for alternatives.
Instead of having to spend nearly 76 working days reading all the digital privacy policies you agree to in a year’s time, according to this research by Aleecia McDonald and Lorrie Franor, what if engagement in understanding them could happen through a game or quiz that improves data and AI literacy? Contextual scenarios could also prompt users to think about underlying social norms and values, and how they are aligned or not with the products and services they use.
You have the option to engage in using a third party tool to negotiate the terms of your user agreement. This will allow you to provide your explicit consent, participate, and directly intervene into the co-constitution of the agreement, including limitations of liability, dispute resolution, and others. As we hope that you will be long-time users of our services, you will continue to have the voice to negotiate as circumstances change. We acknowledge that agreement to our services is not perpetual and we practice continuous and active involvement in how you would like to engage with our services.
Offering users the opportunity to negotiate the terms of agreement when using an AI product or service will inevitably introduce friction in their interaction. However, when anticipated, such friction could improve the interaction and build trust through more meaningful forms of participation, mutual assent, and actual choice in agreeing to contractual terms.
If you decide that you do not want to engage in negotiating the terms of your user agreement and/or would like to opt-out or refuse any specific term, we will allow for your voice to intervene on the reasons why. This will provide a generative feedback loop to help us improve our systems. We will also make available a forum ____ which aims to repair and reconcile harms that are experienced. We will explicitly request your consent that any information gathered from this forum could be leveraged towards algorithmic bug bounty programs and algorithmic audits including community-led efforts.
Thus, the suggested terms above speak to moving from transactional towards relational interactions with contractual agreements that center equity, inclusion, meaningful participation, and building long-term trust relationships.
Conclusion
In summary, as advanced AI models are constantly evolving and put into production, there is a need to open new avenues for a growing ecosystem of third-party actors to engage in building socio-technical safety guardrails. By legitimizing new models for engagement and participation in user agreements, technology companies could signal to their users that they are proactive in their approach to risks and harms of AI, laying the grounds for community-driven and justice-oriented models of AI governance. Thus, actionable forms of AI transparency need to incorporate human-centered user agreements that include: (1) contextual disclosure of data and AI governance, (2) contestability mechanisms and third-party oversight in incident reporting, and (3) engagement and active co-design of user agreements.
This blog post is an update for our ongoing cross-disciplinary collaboration together with Dr. Megan Ma and Dr. Renee Shelby. You can find more information here and please let us know what you think are the biggest challenges and opportunities in evolving human-centered user agreements in building trustworthy AI systems - [email protected].